Why Organizations Face Challenges in Implementing AI.
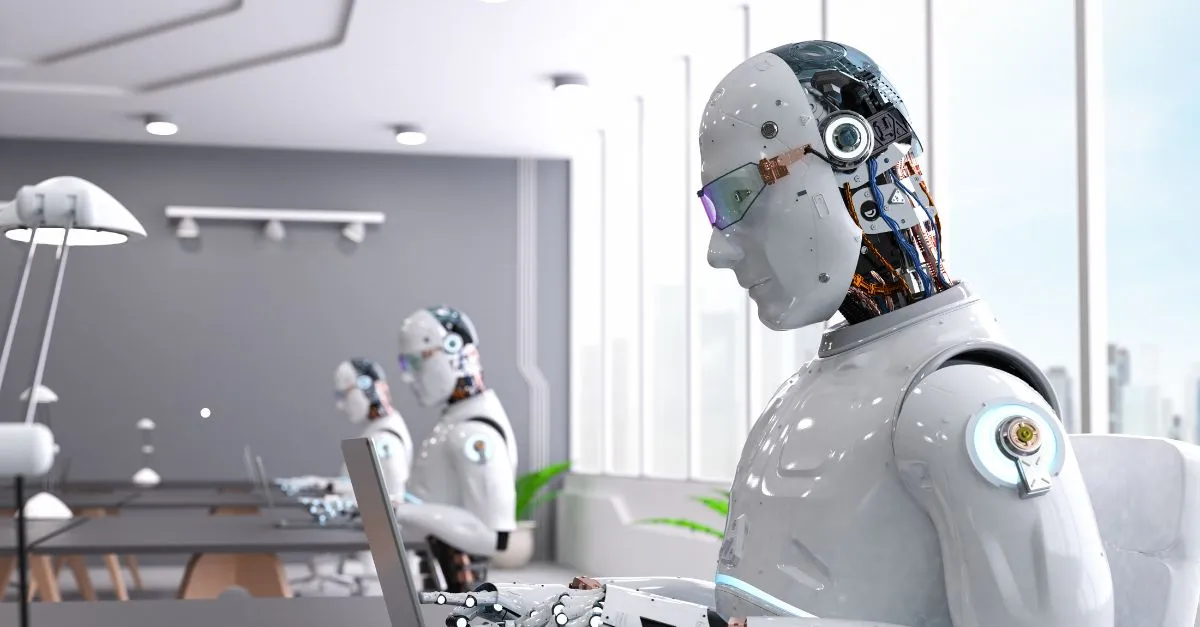
Many are Rushing to Enhance Business Efficiency with AI, but Deploying it Proves More Complex than Expected by Companies.
What's taking place?
Many organizations hold high hopes that AI and machine learning projects will boost revenue by enhancing product/service quality, improving customer satisfaction, accelerating innovation, and more.
Surprisingly, a third of survey respondents are using AI primarily to cut costs by identifying and resolving operational and IT inefficiencies.
However, there's a significant catch: Despite the optimism about AI's potential, most organizations are not fully optimized for its implementation.
The primary hurdle, as per the report, is data management.
Let's delve into this issue: AI relies on substantial volumes of data. Companies do possess such data, but it often exists in various formats – ranging from structured and real-time data to semi-structured IT system data and unstructured rich media data.
Dealing with these diverse data sets poses a considerable standardization challenge. Additionally, many organizations struggle with inadequate IT infrastructure and data governance, making the path to AI deployment even more challenging.
Beyond data, companies also identify compute performance (i.e., how quickly and efficiently processors handle calculations and tasks) and security as significant obstacles to their AI endeavors.
Moreover, the challenges don't end when transitioning to production environments designed for large datasets during the deployment phase; this presents its own set of difficulties.
However, There's More to Consider
AI Brings Environmental Concerns to the Forefront.
According to Stanford's AI Index Report, the deployment of Chat GPT-3 resulted in over 500 tonnes of CO2 emissions in 2022. To put this in context, the average human generates 5.51 tonnes of CO2 in a year.
This raises a critical question for companies with sustainability goals: Can they reconcile these goals with their innovation needs? An experiment conducted by Google's DeepMind team suggests they can.
At two experimental locations, the team achieved energy savings of 9% and 13%, respectively, by using AI to manage commercial cooling systems.
The report proposes that when applied to AI deployment, companies equipped with modern data architecture can mitigate the negative impacts on sustainability performance.
In essence, while AI training poses environmental challenges, companies with the right infrastructure can minimize these effects.
How About the Issue of Skilled Personnel?
On one side lies the data, and on the other, the workforce.
According to a 2022 survey by MIT Technology Review Insights, for companies with revenues ranging from $1 to $500 billion, the most significant challenge in AI deployment is the recruitment of skilled professionals.
Simply having AI and machine learning experts isn't sufficient; organizations require individuals who can bridge the gap between business requirements and technical solutions – these are the translators.
Furthermore, even for roles unrelated to AI, businesses should involve them, as their insights can prove invaluable in the expansion of these projects
Aleksander Mądry, the director of the MIT Center for Deployable Machine Learning, emphasized, "When implementing machine learning, it's not solely about establishing technical trust in the system as an engineer. It's also about ensuring that the people who use it trust it. This entails providing guidance and empowerment, enabling them to verify predictions or intervene when they perceive issues.
TL;DR
To succeed in the AI deployment race, two essential factors must be in place.
Firstly, prioritize data, not only having it but also establishing the necessary infrastructure for data collection and standardization.
Secondly, invest in acquiring skilled talent.
Thoughtful investments in both of these areas will distinguish successful companies from the rest.